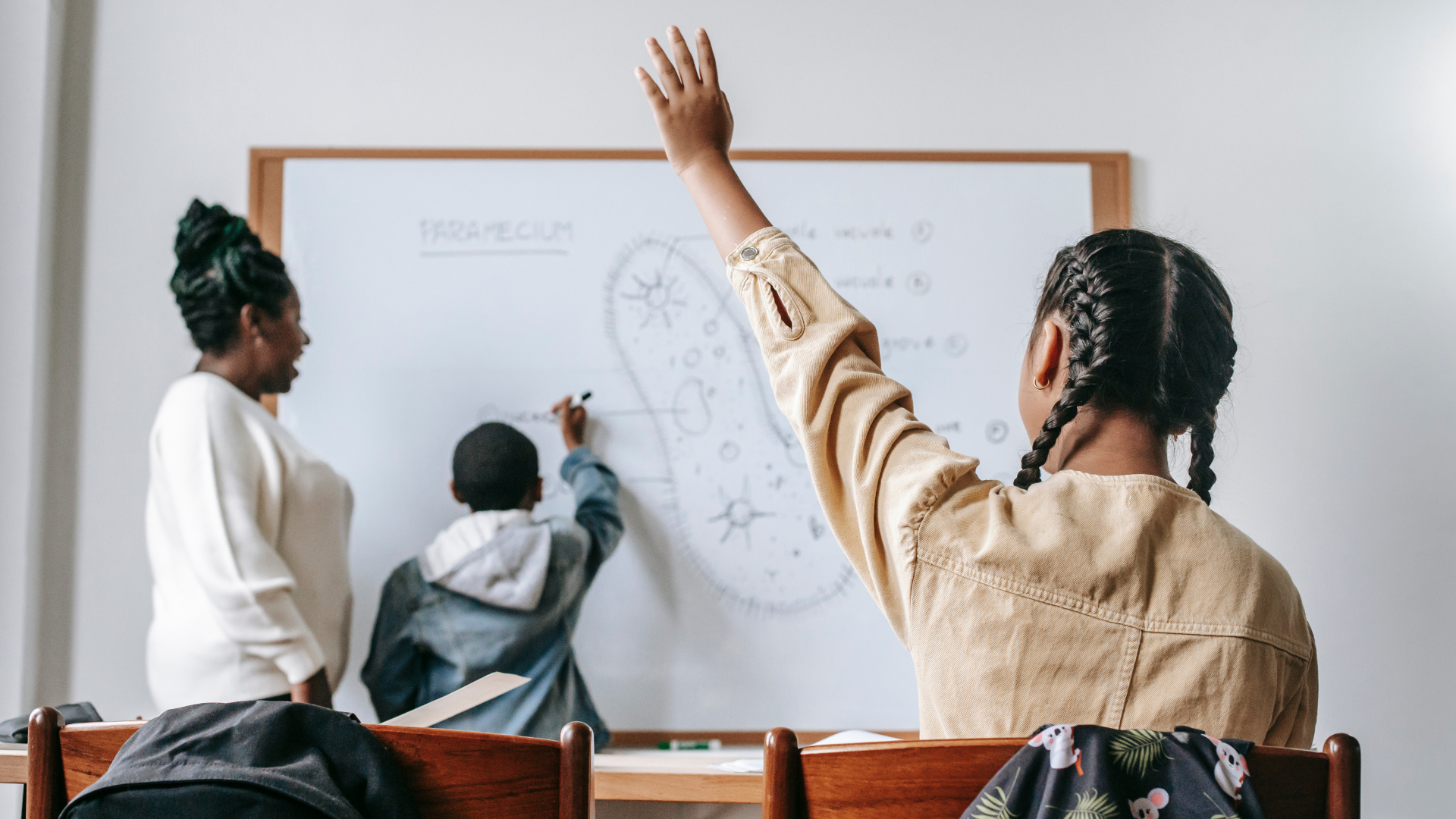
What Makes a Good Science Question: Key Characteristics and Examples
A good science question drives inquiry and exploration, seeking to uncover insights about the world. Specificity is key; the best questions are clearly defined, allowing for focused investigation and meaningful results. This clarity helps scientists avoid ambiguity and narrow their studies to manageable scopes.
Effective science questions often start with “how,” “why,” or “what,” inviting detailed examination rather than simple yes or no answers. This approach encourages critical thinking and deeper analysis, which are essential in scientific research. A well-crafted question not only piques curiosity but also aligns with existing knowledge, enabling researchers to build on previous findings.
Ultimately, the quality of a scientific question can significantly influence the direction and success of research. By honing in on precise, relevant topics, scientists position themselves to make impactful discoveries that contribute to a broader understanding of their field.
Crafting a Scientific Question
A well-crafted scientific question serves as the foundation for effective research. Key elements include clarity, specificity, and objectivity. These components enhance the relevance and applicability of the inquiry across various scientific domains.
Importance of Specificity
Specificity is crucial when formulating a scientific question. It narrows the focus, making it easier to design an experiment or study. A specific question precisely defines the variables involved.
For instance, instead of asking, “How do plants grow?” one might ask, “What is the effect of varying soil ph on the growth rate of tomato plants?” This question pinpoints the specific plant type and variable being tested.
Clear definitions of terms and parameters help eliminate ambiguity, ensuring that the question is easy to understand and address. A precise question facilitates more targeted research, yielding measurable and applicable results.
Avoiding Bias
Bias must be avoided in scientific questioning to ensure objectivity. Questions should encourage impartial investigation, free from preconceived notions or assumptions. Biased questions can lead researchers to seek only evidence that supports their hypothesis.
For example, a question such as “Why does X feature make Y species less successful?” implies a predetermined conclusion. A more neutral approach, like “How does X feature influence the success of Y species?” invites a balanced examination.
Employing standardised terminology and frameworks can also reduce bias. Keeping questions open-ended allows for holistic exploration, leading to genuine scientific inquiry.
Applying to Various Scientific Fields
Crafting effective questions applies across diverse scientific fields such as biology, physics, chemistry, and geology. Each discipline may require specific technical language and parameters.
In biology, researchers might focus on ecological interactions, formulating questions like “What impact does urbanisation have on native bird populations?” In chemistry, a relevant inquiry could be, “How does temperature affect the rate of a chemical reaction?”
Physics might involve questions such as “How does gravitational force vary with the distance between two masses?” Geology could lead to inquiries like “What are the effects of climate change on erosion rates in coastal areas?”
Each example shows the flexibility of well-crafted scientific questions across different contexts, enhancing understanding and facilitating further research.
The Structure of Scientific Inquiry
The structure of scientific inquiry consists of systematic approaches to explore ideas and concepts. It emphasises the importance of the scientific method and the formulation of testable hypotheses to ensure the reliability of research findings.
Utilising the Scientific Method
The scientific method provides a structured framework for investigation. It typically involves several key steps:
- Observation: Begin with careful observation of phenomena.
- Question: Formulate a question based on initial observations.
- Research: Conduct background research to gather existing information.
- Hypothesis: Develop a testable hypothesis.
- Experiment: Design and perform experiments to test the hypothesis.
- Analysis: Analyse the data collected.
- Conclusion: Draw conclusions based on the data, determining if the hypothesis is supported.
This method allows for a rigorous approach to inquiry, making findings replicable and verifiable.
Developing a Testable Hypothesis
A hypothesis is a critical component of scientific research. It presents a clear, testable statement predicting outcomes based on observations.
Key aspects of a robust hypothesis include:
- Clarity: It must be precise and unambiguous.
- Testability: It should be possible to test the hypothesis through experimentation.
- Variables: Identify independent and dependent variables to establish relationships.
For example, if a researcher observes that plants grow differently in varied light, they might hypothesise that “increased light exposure leads to faster growth in plants.” This statement can then be tested through controlled experiments to confirm or refute the hypothesis.
Experimentation and Data Analysis
Effective experimentation and data analysis are foundational to formulating quality science questions. A well-designed experiment accurately addresses the research question, while thorough data analysis helps draw reliable conclusions from the results.
Designing a Robust Experiment
Designing an experiment involves several crucial steps. First, clearly define the hypothesis to test. This forms the basis for the experimental structure.
Next, choose an appropriate method. This may involve a controlled environment or field study. Define the sample size and ensure it is large enough for validity.
The design must also incorporate control groups to eliminate bias. Identifying the dependent and independent variables is essential. For instance, in a plant growth study, light would be the independent variable, while growth rate would be the dependent variable.
Managing Variables
Control of variables is vital to ensure valid results. Independent variables are intentionally manipulated, while dependent variables are measured to observe effects.
Extraneous variables must be managed to prevent interference with results. This can involve randomisation or standardisation of conditions.
An example is ensuring that all plants receive equal watering. Identifying potential confounding variables early reduces the risk of flawed conclusions. They can often skew data and lead to incorrect inferences about relationships.
Analysing Results
After conducting experiments, data analysis plays a crucial role. Quantitative data can be analysed using statistical tests to determine significance levels. Common tests include t-tests and ANOVA.
Data should be presented clearly, using graphs and tables where appropriate. This enhances understanding of trends and relationships.
Qualitative data analysis may involve thematic analysis or coding to identify patterns. Drawing conclusions requires careful consideration of results alongside predictions made before the experiment.
Ultimately, robust experimentation and thorough data analysis lead to credible insights, informing further research or practical applications.
Comments (0)